
View from the Chinese polar research icebreaker Xuelong (“Snow Dragon”) – image courtesy the researchers
story by Helen Hill for MITgcm
MITgcm underpins a new Chinese Arctic sea-ice prediction system.
The recent dramatic reduction and thinning of summertime ice cover is opening up Arctic navigation to commercial and scientific shipping, and this uptick in maritime activity has prompted efforts to improve operational sea-ice forecasting. In response, a team of Chinese researchers has developed ArcIOPS (the Arctic Ice Ocean Prediction System), an ensemble-based modeling tool for operational Arctic sea ice forecasting. At the heart of ArcIOPS is a regional Arctic configuration of the MITgcm.
ArcIOPS sea-ice forecast capabilities were field-tested during a Chinese National Arctic Research Expedition in summer 2017 when they were used for the first time operationally by the Chinese polar research icebreaker Xuelong (“Snow Dragon”). An assessment of how ArcIOPS predictions stacked up was recently published in the Journal of Glaciology.
Driving its sea-ice – ocean coupled model with weather forecasts from NCEP’s Global Forecast System, ArcIOPS uses a localized error subspace transform ensemble Kalman filter to assimilate weekly merged CryoSat-2 and Soil Moisture and Ocean Salinity sea-ice thickness data together with daily Advanced Microwave Scanning Radiometer 2 (AMSR2) sea-ice concentration data.
The ocean and sea-ice components of ArcIOPS are based on the MITgcm sea-ice – ocean model, a regional configuration with a horizontal resolution of ∼18 km and an open boundary around 55 N°. The sea-ice model uses viscous-plastic dynamics and zero-layer thermodynamics. To parameterize thick ice growth in the sub-grid scale, the team use seven thickness categories with a prescribed homogeneous distribution between zero and twice the mean ice floe thickness, applying the same approach to snow. See the paper for full model details.
Evaluating the forecast sea-ice concentration against AMSR2 and Special Sensor Microwave Imager/Sounder sea-ice concentration data the team (which comprised researchers Longjiang Mu, Xi Liang, Qinghua Yang, Jiping Liu, and Fei Zheng) found that, when compared to the in-situ observations and predictions of the Pan-Arctic Ice-Ocean Modeling and Assimilation System, with the exception of the Beaufort Gyre, the ArcIOPS predicted sea-ice thickness well.
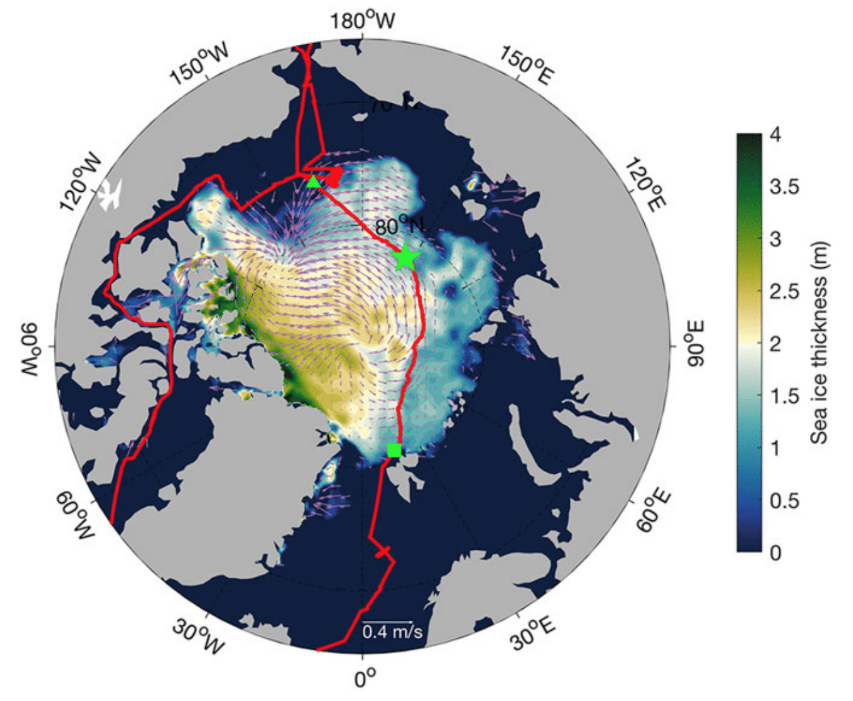
72 hour forecasts of sea-ice thickness and drift on 9 August 2017. The red curve shows Xuelong’s trajectory during CHINARE2017 – Mu et al 2019
Corresponding author Qinghua says a second version of ArcIOPS, which seeks to address the weaknesses observed in the Beaufort sector by including sea surface temperature assimilation is under development and will be put into operational use in the near future.
To find out more about this work email Qinghua.
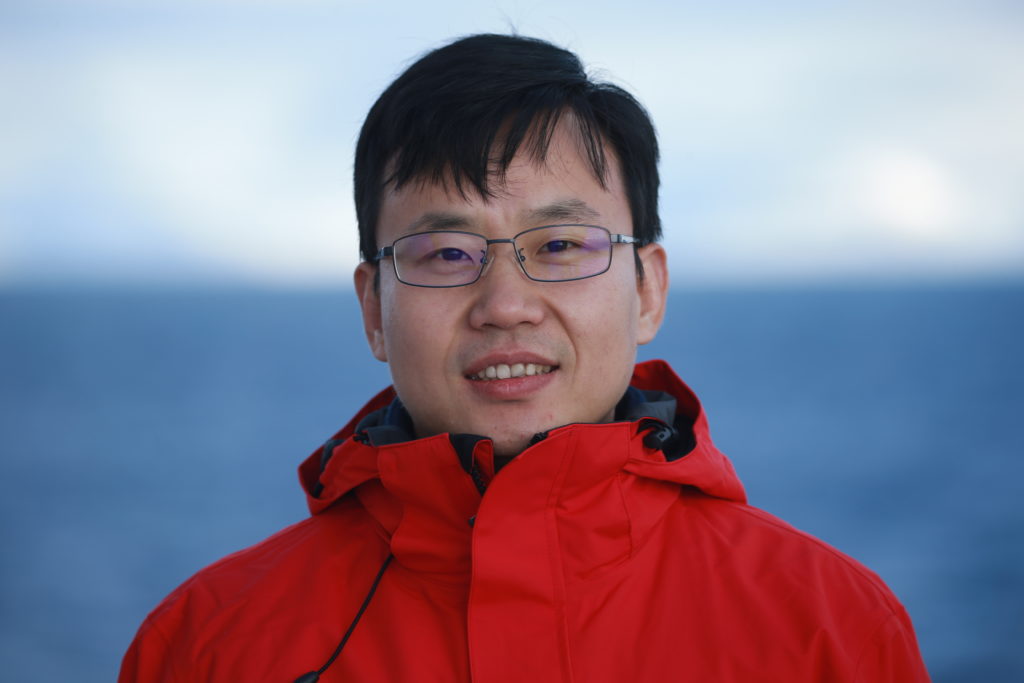
Qinghua Yang works at Sun Yat-sen University and Southern Marine Science and Engineering Guangdong Laboratory (Zhuhai). He began using MITgcm in 2009. “With MITgcm, my colleagues and I built the first Chinese Arctic Sea Ice Prediction System in 2010 and since then have continued to use MITgcm in Arctic and Antarctic sea ice-ocean numerical modeling, data assimilation, and prediction.” During a stint at AWI between 2013 and 2015 Qinghua met and began working closely with fellow long-time MITgcmer Martin Losch, subsequently co-authoring multiple papers with him. When he’s not busy trying to figure out how thick the ice will be, Qinghua says me likes to go to the Arctic and Antarctic and has been there four times. “I have spent two winters at the Antarctic Great Wall station. I really enjoy the peace and beauty there,” he says.
This Month’s Featured Publication
- Mu L, Liang X, Yang Q, Liu J, Zheng F (2019), Arctic Ice Ocean Prediction System: evaluating sea-ice forecasts during Xuelong’s first trans-Arctic Passage in summer 2017, Journal of Glaciology, doi: 10.1017/jog.2019.55
Related Publications
This is actually the second time MITgcm has covered work involving Qinghua. The first time was in our 2011 post 北极海冰数值预报的初步研究!!!MITgcm基于海冰!海洋耦合模式!”# $ Other papers include:
Yang, Q., Liu, J., Zhang, Z., Wu, H., Li, Q., Xing, J., A preliminary study of the Arctic sea ice numerical forecasting: coupled sea ice–ocean modelling experiments based on MITgcm, Chinese J. Atmos. Sci., 2011, 35(3), 473–482 [in Chinese]
Yang, Q., Li, C., Xing, J., Li, Q., Zhang, L. Li, M. (2012), Arctic sea ice forecasting experiments in the summer of 2010, Chinese J. Polar Res.(2012) 24(1), 87–94 [in Chinese]
Yang, Q., Losa, S.N., Losch, M., Tian-Kunze, X., Nerger, L., Liu, J., Kaleschke, L., Zhang, Z. (2014), Assimilating SMOS sea ice thickness into a coupled ice-ocean model using a local SEIK filter, Journal of Geophysical Research-Oceans, doi: 10.1002/2014JC009963
Yang, Q.,Liu, J., Zhang, Z., Sui, C., Xing, J., Li, M., Li, C., Zhao, J., Zhang, L. (2014), Sensitivity of the Arctic sea ice concentration forecasts to different atmospheric forcing: a case study, Acta Oceanologica Sinica, doi: 10.1007/s13131-014-0566
Yang, Q. , Losa, S.N., Losch, M., Jung, T., Nerger, L. (2015), The role of atmospheric uncertainty in Arctic sea ice data assimilation and prediction, Quarterly Journal of the Royal Meteorological Society, doi: 10.1002/qj.2523
Yang, Q., Losa, S.N., Losch, M., Liu, J., Zhang, Z., Nerger, L., Yang, H. (2015), Assimilating summer sea ice concentration into a coupled ice-ocean model using a localized SEIK filter, Annals of Glaciology, doi: 10.3189/2015AoG69A740
Yang, Q., Losch, M., Losa, S.N., Jung, T., Nerger, L., Lavergne, T. (2016), The challenge and benefit of using sea ice concentration satellite data products with uncertainty estimates in summer sea ice data assimilation, The Cryosphere, doi: 10.5194/tc-10-1-2016
Yang, Q., Losch, M., Losa, S.N., Jung, T., Nerger, L. (2016), Taking into account atmospheric uncertainty improves sequential assimilation of SMOS sea ice thickness data in an ice-ocean model, Journal of Atmospheric and Oceanic Technology, doi: 10.1175/JTECH-D-15-0176.1
Liang, X., Yang, Q., Nerger, L., Losa, S., Zhao, Z., Zheng, F., Zhang, L., Wu, L. (2017), Assimilating Copernicus SST data into a pan-Arctic ice-ocean coupled model with a local SEIK filter, Journal of Atmospheric and Oceanic Technology, doi: 10.1175/JTECH-D-16-0166.1
Mu, L., Yang, Q., Losch, M., Losa, S., Ricker, R., Nerger, L., Liang, X. (2018), Improving sea ice thickness estimates by assimilating CryoSat-2 and SMOS sea ice thickness data simultaneously, Quarterly Journal of the Royal Meteorological Society, doi: 10.1002/qj.3225
Mu, L., Losch, M., Yang, Q., Ricker, R., Losa, S., Nerger, L. (2018), Arctic-wide sea-ice thickness estimates from combining satellite remote sensing data and a dynamic ice-ocean model with data assimilation during the CryoSat-2 period, Journal of Geophysical Research-Oceans, doi: 10.1029/2018JC014316
Yang, Q., Mu, L., Wu, X., Liu, J., Zheng, F., Zhang, J., Li, C. (2019), Improving Arctic sea ice seasonal outlook by ensemble prediction using an ice-ocean model, Atmospheric Research, doi: 10.1016/j.atmosres.2019.04.021
Min, C., Mu, L., Yang, Q., Ricker, R., Shi, Q., Han, B., Wu, R., Liu, J. (2019), Sea ice export through the Fram Strait derived from a combined model and satellite data set, The Cryosphere Discuss., doi: 10.5194/tc-2019-157
Liang, X., Losch, M., Nerger, L., Mu, L., Yang, Q., Liu, C. (2019), Using sea surface temperature observations to constrain upper ocean properties in an Arctic sea ice-ocean data assimilation system, Journal of Geophysical Research-Oceans, doi: 10.1029/2019JC015073
Other New Publications this Month
Katherine Adams, Jennifer MacKinnon, Andrew J. Lucas, Jonathan Nash, Emily Shroyer, J. Thomas Farrar (2019), Multi-platform observations of small-scale lateral mixed layer variability in the northern Bay of Bengal, Deep Sea Research Part II: Topical Studies in Oceanography, doi: 10.1016/j.dsr2.2019.07.017
Sultan Albarakati, Ricardo M.Lima, Loïc Giraldi, Ibrahim Hoteit, Omar Knio (2019), Optimal 3D trajectory planning for AUVs using ocean general circulation models, Ocean Engineering, doi: 10.1016/j.oceaneng.2019.
Thomas Bolton, Ryan Abernathey, Laure Zanna (2019), Regional and Temporal Variability of Lateral Mixing in the North Atlantic, Journal of Physical Oceanography, doi: 10.1175/JPO-D-19-0042.1
Thiago Dias dos Santos (2019), Adaptive Mesh Refinement Applied to Marine Ice Sheet Simulation, Thesis presented to the School of Civil Engineering, University of Campinas, Brazil Doctoral Dissertation (pdf)
A. R. Gorbushkin, A. N. Demidov (2019), Variability of Thermohaline Characteristics at 26.5° N in Reanalyses and Oceanographic Section Data, Russian Meteorology and Hydrology, doi: 10.3103/S1068373919070069
Bing Han, Carsten Eden (2019), The energetics of internal tides at the Luzon Ridge, Ocean Dynamics, doi: 10.1007/s10236-019-01297-9
Bruce M. Howe et al (2019), SMART Cables for Observing the Global Ocean: Science and Implementation, Frontiers in Marine Science, doi: 10.3389/fmars.2019.00424
Isabela Alexander-Astiz Le Bras, Maike Sonnewald, John M. Toole (2019), A barotropic vorticity budget for the subtropical North Atlantic based on observations, Journal of Physical Oceanography, doi: 10.1175/JPO-D-19-0111.1
Guancheng Li, Yuhong Zhang, Jingen Xiao, Xiangzhou Song, John Abraham, Lijing Cheng, Jiang Zhu (2019), Examining the salinity change in the upper Pacific Ocean during the Argo period, Climate Dynamics, doi: 10.1007/s00382-019-04912-z
Chao Liu, Xinfeng Liang, Rui M. Ponte, Nadya Vinogradova, and Ou Wang (2019), Vertical redistribution of salt and layered changes in global ocean salinity, Nature Communications, doi: 10.1038/s41467-019-11436-x
Yafei Nie, Yuzhe Wang, and Xianqing Lv (2019), Acquiring the Arctic-scale spatial distribution of snow depth based on AMSR-E snow depth product, Journal of Atmospheric and Oceanic Technology, doi: 10.1175/JTECH-D-18-0217.1
Sivareddy Sanikommu, Deep Sankar, Benerjee Balaji, Baduru Biswamoy, Paul Arya, Paul, Kunal Chakraborty, Ibrahim Hoteit (2019), Impact of Dynamical Representational Errors on an Indian Ocean Ensemble Data Assimilation System, Quarterly Journal of the Royal Meteorological Society, doi: 10.1002/qj.3649
Do you have news about research using MITgcm? We are looking for contributions to these pages. If you have an interesting MITgcm project (ocean, atmosphere, sea-ice, physics, biology or otherwise) that you want to tell people about, get in touch. To make a post, contact Helen